AI Assistant Analytics: Implementation and Monitoring - A Comprehensive Guide
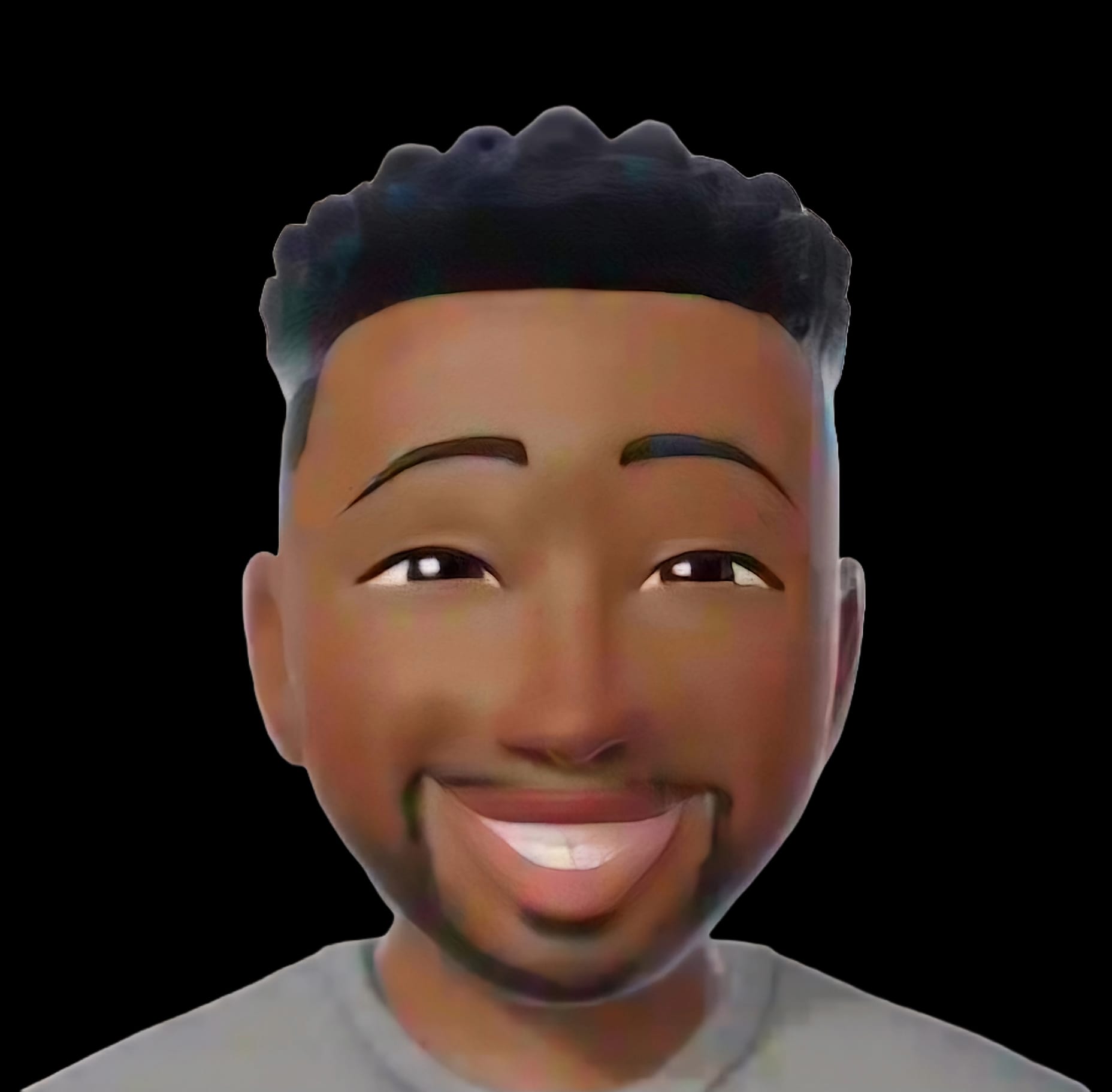
Paa Yaw
18 Nov 2024

In today's digital landscape, implementing and monitoring AI assistant analytics has become crucial for businesses seeking to optimise their conversational AI solutions. This comprehensive guide explores the intricate aspects of analytics implementation, monitoring strategies, and best practices for maximising AI assistant performance.
Understanding AI Assistant Analytics
At its core, AI assistant analytics involves collecting, analysing, and interpreting data from conversational AI interactions. This encompasses user engagement metrics, conversation flow analysis, response accuracy, and user satisfaction indicators. The implementation of robust analytics systems enables organisations to make data-driven decisions and continuously improve their AI assistants.
Implementation Framework
The foundation of effective AI assistant analytics begins with a well-structured implementation framework. This involves establishing clear objectives and identifying key performance indicators (KPIs) that align with business goals. Essential metrics typically include conversation completion rates, user satisfaction scores, response times, and resolution rates.
Data Collection Architecture
Implementing a robust data collection system requires careful consideration of various technical components. Modern AI assistant platforms should incorporate event tracking, conversation logging, and user interaction monitoring. This data architecture must be designed to capture both structured and unstructured data while maintaining data privacy and security compliance.
Real-time Monitoring Systems
Real-time monitoring capabilities are essential for maintaining optimal AI assistant performance. Advanced monitoring systems should track conversation flows, detect anomalies, and alert administrators to potential issues. This includes monitoring response latency, error rates, and user sentiment in real-time.
Performance Metrics Analysis
Comprehensive analytics implementation must include detailed performance metric analysis. This involves examining:
Response Accuracy: Measuring the precision of AI responses and understanding areas for improvement.
Conversation Flow Efficiency: Analysing the smoothness of dialogue transitions and identifying bottlenecks.
User Engagement Levels: Understanding how users interact with the AI assistant and their engagement patterns.
Resolution Rates: Tracking successful query resolutions and identifying common failure points.
Integration with Business Intelligence
Effective AI assistant analytics should integrate seamlessly with existing business intelligence systems. This integration enables organisations to correlate AI assistant performance with broader business metrics, providing valuable insights for strategic decision-making.
Continuous Improvement Framework
Implementing a continuous improvement framework is crucial for long-term success. This involves:
Regular Performance Reviews: Conducting systematic analyses of AI assistant performance metrics.
Feedback Loop Implementation: Establishing mechanisms for collecting and acting on user feedback.
Model Refinement: Using analytics insights to improve AI models and conversation flows.
Security and Compliance Considerations
When implementing AI assistant analytics, security and compliance must be prioritised. This includes ensuring data protection, implementing access controls, and maintaining compliance with relevant regulations such as GDPR and Australian Privacy Principles.
Advanced Analytics Features
Modern AI assistant analytics should incorporate advanced features such as:
Sentiment Analysis: Understanding user emotions and satisfaction levels.
Conversation Pattern Recognition: Identifying common user behaviours and interaction patterns.
Predictive Analytics: Anticipating user needs and potential issues before they arise.
Monitoring Best Practices
Effective monitoring requires establishing clear protocols and responsibilities. This includes:
Setting Up Alert Thresholds: Defining acceptable performance ranges and alert triggers.
Creating Response Protocols: Establishing procedures for addressing performance issues.
Regular Reporting: Implementing structured reporting schedules for stakeholder communication.
Future-Proofing Analytics Systems
As AI technology evolves, analytics systems must be designed with scalability and adaptability in mind. This includes planning for future integration capabilities, emerging analytics tools, and evolving business requirements.
Ready to implement advanced analytics for your AI assistant? Click here to schedule your free consultation with Nexus Flow Innovations and discover how our expertise can help you build and monitor high-performing conversational AI solutions.
Keywords: AI assistant analytics, conversational AI monitoring, analytics implementation, performance metrics, real-time monitoring, business intelligence integration, continuous improvement, sentiment analysis, conversation analytics, AI performance optimization, data collection architecture, analytics best practices, AI assistant metrics, conversation flow analysis, user engagement analytics, AI monitoring systems, analytics implementation framework, conversation pattern recognition, predictive analytics, AI assistant performance